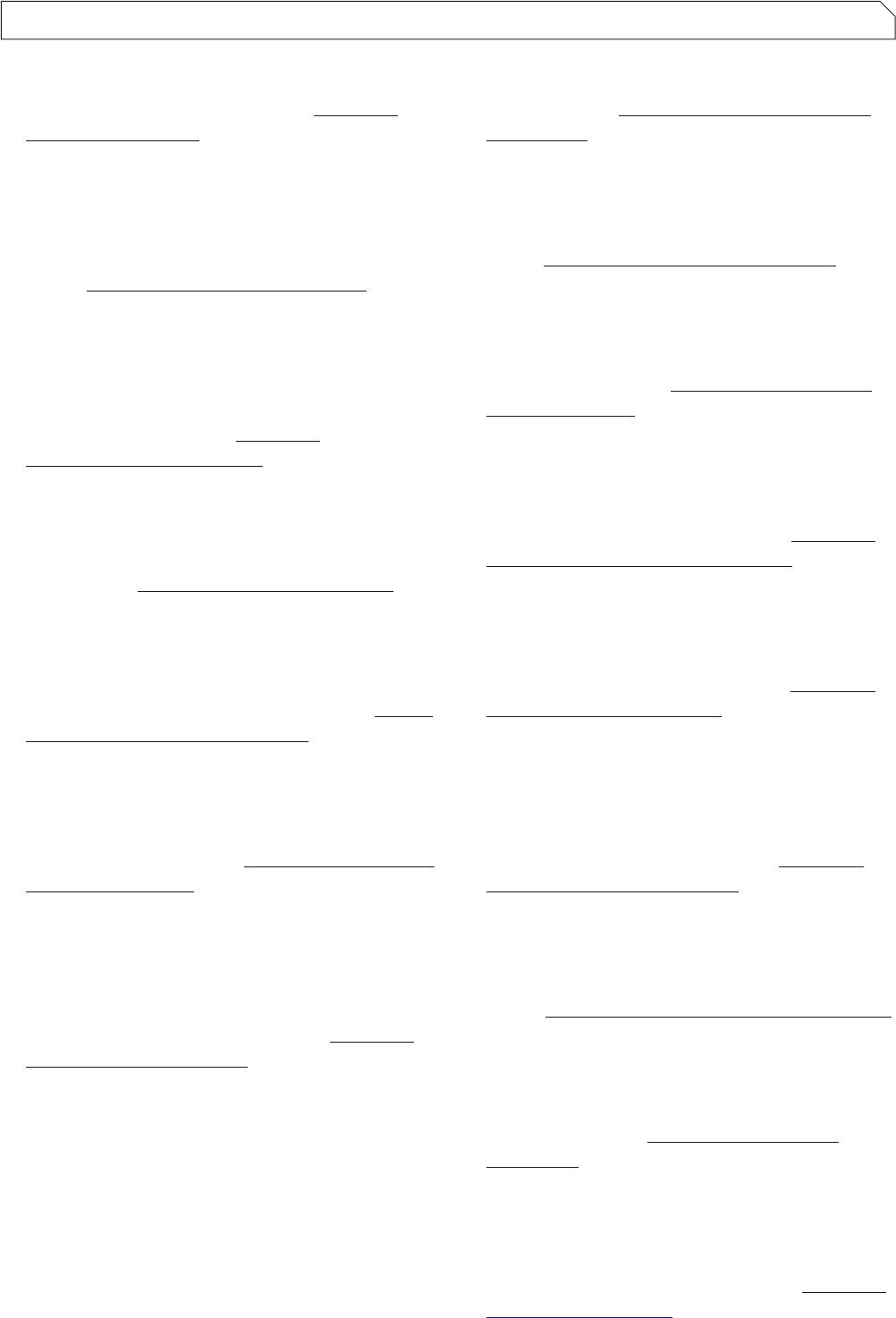
D. Mishra et al., J. Spectral Imaging 10, a1 (2021) 11
AIP Conf. Proc. 283,192–201(1993).hps://doi.
org/10.1063/1.44433
21.
G.Zuendorf,N.Kerrouche,K.HerholandJ.C.
Baron,“Ecientprincipalcomponentanalysisfor
mulvariate3Dvoxel-basedmappingofbrain
funconalimagingdatasetsasappliedtoFDG-PET
andnormalaging”,Hum. Brain Mapp. 18(1),13–21
(2003).hps://doi.org/10.1002/hbm.10069
22.
C. Rodarmel and J. Shan, “Principal component anal-
ysisforhyperspectralimageclassicaon”,Survey.
Land Inform. Sci. 62(2), 115 (2002).
23.
M.S. Tooms, Colour Reproducon in Electronic Imaging
Systems: Photography, Television, Cinematography.
John Wiley & Sons (2016). hps://doi.
org/10.1002/9781119021780
24.
A.A. Green, M. Berman, P. Switzer and M.D. Craig,
“Atransformaonfororderingmulspectraldatain
termsofimagequalitywithimplicaonsfornoise
removal”,IEEE Trans. Geosci. Remote Sens. 26(1),
65–74(1988).hps://doi.org/10.1109/36.3001
25.
S.Kiyotoki,J.Nishikawa,T.Okamoto,K.Hamabe,M.
Saito, A. Goto, Y. Fujita, Y. Hamamoto, Y. Takeuchi,
S.SatoriandI.Sakaida,“Newmethodfordetecon
ofgastriccancerbyhyperspectralimaging:apilot
study”,J. Biomed. Opt. 18(2),026010(2013).h ps: //
doi.org/10.1117/1.JBO.18.2.026010
26.
G.Lu,D.Wang,X.Qin,S.Muller,J.V.Lile,X.Wang,
A.Y. Chen, G. Chen and B. Fei, “Histopathology
featureminingandassociaonwithhyperspectral
imagingforthedeteconofsquamousneoplasia”,
Sci. Rep. 9(1),17863(2019).hps://doi.org/10.1038/
s41598-019-54139-5
27.
A.Pardo,J.A.Guérrez-Guérrez,I.Lihacova,J.M.
López-HigueraandO.M.Conde,“Onthespectral
signatureofmelanoma:anon-parametricclassi-
caonframeworkforcancerdeteconinhyper-
spectralimagingofmelanocyclesions”,Biomed.
Opt. Express 9(12),6283–6301(2018).hps://doi.
org/10.1364/BOE.9.006283
28.
X. Hadoux, F. Hui, J.K.H. Lim, C.L. Masters, A.
Pébay,S.Chevalier,J.Ha,S.Loi,C.J.Fowler,C.
Rowe,V.L.Villemagne,E.N.Taylor,C.Fluke,J.-P.
Soucy,F.Lesage,J.-P.Sylvestre,P.Rosa-Neto,S.
Mathotaarachchi,S.Gauthier,Z.S.Nasreddine,
J.D.Arbour,M.-A.Rhéaume,S.Beaulieu,M.
Dirani,C.T.O.Nguyen,B.V.Bui,R.Williamson,J.G.
CrowstonandP.vanWijngaarden,“Non-invasivein
vivohyperspectralimagingoftherenaforpotenal
biomarkeruseinAlzheimer’sdisease”,Nat. Commun.
10,4227(2019).hps://doi.org/10.1038/s41467-
019-12242-1
29.
S.Ortega,H.Fabelo,D.K.Iakovidis,A.Koulaouzidis
andG.M.Callico,“Useofhyperspectral/mulspec-
tral imaging in gastroenterology. shedding some-
dierent-lightintothedark”,J. Clin. Med. 8(1),36
(2019).hps://doi.org/10.3390/jcm8010036
30.
S.J. Leavesley, M. Walters, C. Lopez, T. Baker, P.F.
Favreau, T.C. Rich, P.F. Rider and C.W. Boudreaux,
“Hyperspectralimaginguorescenceexcitaon
scanningforcoloncancerdetecon”,J. Biomed. Opt.
21(10),104003(2016).hps://doi.org/10.1117/1.
JBO.21.10.104003
31.
E.Kho,L.L.deBoer,K.K.VandeVijver,F.van
Duijnhoven,M.VranckenPeeters,H.Sterenborg
andT.J.M.Ruers,“Hyperspectralimagingforresec-
onmarginassessmentduringcancersurgery”,Clin.
Cancer Res. 25(12),3572–3580(2019).hps://doi.
org/10.1158/1078-0432.CCR-18-2089
32.
T. Du, D.K. Mishra, L. Shmuylovich, A. Yu, H.
Hurbon, S.T. Wang and M.Y. Berezin, “Hyperspectral
imagingandcharacterizaonofallergiccon-
tactdermasintheshort-waveinfrared”,J.
Biophoton. 13(9), e202000040 (2020). hps://doi.
org/10.1002/jbio.202000040
33.
M.Lukina,K.Yashin,E.Kisileva,A.Alekseeva,
V.Dudenkova,E.V.Zagaynova,E.Bederina,I.
Medyanik, W. Becker, D. Mishra, M.Y. Berezin,
V.I.ShcheslavskiyandM.Shirmanova,“Label-free
macroscopicuorescencelifemeimagingofbrain
tumors”,Front. Oncol. in press (2021). hps://doi.
org/10.3389/fonc.2021.666059
34.
A.Lowe,N.HarrisonandA.P.French,
“Hyperspectralimageanalysistechniquesforthe
deteconandclassicaonoftheearlyonsetof
plantdiseaseandstress”,Plant Meth. 13(1), 80
(2017).hps://doi.org/10.1186/s13007-017-0233-z
35.
P.V.Manley,V.Sagan,F.B.FritschiandJ.G.Burken,
“Remotesensingofexplosives-inducedstressin
plants:hyperspectralimaginganalysisforremote
deteconofunexplodedthreats”,Remote Sens.
11(15),1827(2019).hps://doi.org/10.3390/
rs11151827
36.
D.M. Kim, H. Zhang, H. Zhou, T. Du, Q. Wu,
T.C.MocklerandM.Y.Berezin,“Highlysensive
image-derivedindicesofwater-stressedplants
using hyperspectral imaging in SWIR and histo-
gramanalysis”,Sci. Rep. 5,15919(2015).hps://doi.
org/10.1038/srep15919